New Algorithm for EEG and EMG Separation
The paper presents newly proposed algorithm for the blind separation of EEG and EMG sources measured by high density electrode arrays. The algorithm is based on the maximization of the variance of variances of filtered principal components. Utilized high pass filter was optimized in order to extract the information which is used by the gradient algorithm to separate New Algorithm for EEG and EMG SeparationEEG and EMG components. The performance of the algorithm was evaluated by its use for the muscular artifacts removal. Present muscular artifacts were extracted from the estimated components with the use of the previously used classifier. It is compared with other similar approaches and it is shown that the suggested algorithm achieves higher quality of the processed EEG signal especially in the case of strong muscular artifacts and is therefore useful for the preprocessing of the EEG records contaminated with the muscle activity.
Keywords:
BSS, variance of variances, gradient, muscular artifacts, EMG, EEG
Autoři:
Jan Šebek; Radoslav Bortel
Působiště autorů:
Dept. of Circuit Theory, Czech Technical University in Prague, Czech Republic
Vyšlo v časopise:
Lékař a technika - Clinician and Technology No. 2, 2015, 45, 43-47
Kategorie:
Původní práce
Souhrn
The paper presents newly proposed algorithm for the blind separation of EEG and EMG sources measured by high density electrode arrays. The algorithm is based on the maximization of the variance of variances of filtered principal components. Utilized high pass filter was optimized in order to extract the information which is used by the gradient algorithm to separate New Algorithm for EEG and EMG SeparationEEG and EMG components. The performance of the algorithm was evaluated by its use for the muscular artifacts removal. Present muscular artifacts were extracted from the estimated components with the use of the previously used classifier. It is compared with other similar approaches and it is shown that the suggested algorithm achieves higher quality of the processed EEG signal especially in the case of strong muscular artifacts and is therefore useful for the preprocessing of the EEG records contaminated with the muscle activity.
Keywords:
BSS, variance of variances, gradient, muscular artifacts, EMG, EEG
Zdroje
[1] BELOUCHRANI, A., ABED-MERAIM, K., CARDOSO, J.-F., MOULINES E. A Blind Source Separation Technique Using Second-Order Statistics, IEEE TRANSACTIONS ON SIGNAL PROCESSING, FEBRUARY 1997, vol. 45, no. 2, p. 434 - 444.
[2] HYVARINEN, A., OJA, E. Independent Component Analysis: algorithms and applications, Neural Networks, 2000, vol. 13, no. 4-5, p. 411-430.
[3] MAMMONE, N., MORABITO, F.C. Enhanced Automatic Artifact Detection based on Independent Component Analysis and Renyi's Entropy. Neural Networks, 2008, vol. 21, p. 1029-1040.
[4] DELORME, A., SEJNOWSKI, T., MAKEIG, S. Enhanced Detection of Artifacts in EEG Data using Higher-order Statistics and Independent Component Analysis. NeuroImage, 2007, vol. 34, p. 1443-1449.
[5] JIANWEI, W. Estimating source kurtosis directly from observation data for ICA. Signal Processing, 2011, vol. 91, p. 1150-1156.
[6] NG, S.-C., RAVEENDRAN, P. Removal of EOG artifacts using ICA regression method. IFMBE Proceedings 21 IFMBE 2008, vol. 1, p. 226-229.
[7] ŠEBEK, J., Classification of the EEG and EMG sources estimated by ICA. 17th INTERNATIONAL STUDENT CONFERENCE ON ELECTRICAL ENGINEERING, 2013, ISBN 978-80-01--05242-6.
[8] ŠEBEK, J., Metody odstraňování svalových artefaktů ze záznamů EEG. IV. Letní doktorandské dny 2014, ISBN 978-80-01-05506-9.
[9] ŠEBEK, J., Removal of Muscular Artifacts from EEG Records. 18th INTERNATIONAL STUDENT CONFERENCE ON ELECTRICAL ENGINEERING, 2014, ISBN 978-80-01-05499-4.
[10] KÁBRT, J., KÁBRT, J. LEXICON MEDICUM. 2nd ed. Galen, Praha, 2004, ISBN 80-7262-235-8.
[11] BORTEL, R., SOVKA, P. Potential approximation in realistic Laplacian computation, Clinical Neurophysiology, 2013, vol. 124, no. 3, p. 462-473.
[12] BORTEL, R., SOVKA, P. Electrode Position Scaling in Realistic Laplacian Computation. IEEE Transactions on Biomedical Engineering, 2008, vol. 55, no. 9, p. 2314 - 2316.
[13] BORTEL, R., SOVKA, P. Statistical evaluation of coherence estimated from optimally beamformed signals, Computers in Biology and Medicine, 2013, vol. 43, no. 9, p. 1286 - 1262.
[14] DELORME, A., SEJNOWSKI, T., MAKEIG, S. Enhanced detection of artifacts in EEG data using higher-order statistics and independent component analysis, NeuroImage, 2007, vol. 34, p. 1443 - 1449.
[15] BLANCO, S., GARCIA, H., QUIAN QUIROGA, R., ROMANELLI, L., ROSSO, O., A. Stationarity of the EEG series, IEEE Eng Med Biol, 1995a, vol. 14, p. 395-399.
[16] BLANKERTZ, B., TOMIOKA, R., LEMM, S., KAWANABE, M., and MULLER, K.-R. Optimizing spatial filters for robust EEG single-trial analysis, IEEE Signal Proc Magazine, 2008, vol. 25, no. 1, p. 41 - 56.
[17] SHWEDYK, E., BALUSUBRAMANIAN, R., and SCOTT, R. N. A non-stationary model for the electromyogram, IEEE Trans. Biomed. Eng, 1977, vol. BME-24, p. 417 - 424.
[18] RUSH, S., DRISCOLL, D. A. Current distribution in the brain from surface electrodes, Anesthesia and Analgesia, 1968, vol. 47, no. 6, p. 717 - 723.
Štítky
BiomedicínaČlánok vyšiel v časopise
Lékař a technika
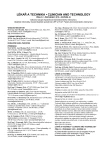
2015 Číslo 2
Najčítanejšie v tomto čísle
- Použití radiochromního gelového dozimetru pro verifikaci dávkové distribuce ve stereotaktické radiochirurgii
- STRUCTURED PDMS USED AS ACTIVE ELEMENT FOR A BIOMIMETICS INSPIRED FLUID TRANSPORTER
- New Algorithm for EEG and EMG Separation
- Detection of facial areas in ThERMAL IMAGES