METHODS FOR AUTOMATIC ESTIMATION OF THE NUMBER OF CLUSTERS FOR K-MEANS ALGORITHM USED ON EEG SIGNAL: FEASIBILITY STUDY
Lots of brain diseases are recognized by EEG recording. EEG signal has a stochastic character, this stochastic nature makes the evaluation of EEG recording complicated. Therefore we use automatic classification methods for EEG processing. These methods help the expert to find significant or physiologically important segments in the EEG recording. The k-means algorithm is a frequently used method in practice for automatic classification. The main disadvantage of the k-means algorithm is the necessary determination of the number of clusters. So far there are many methods which try to determine optimal number of clusters for k-means algorithm. The aim of this study is to test functionality of the two most frequently used methods on EEG signals, concretely the elbow and the silhouette method. In this feasibility study we compared the results of both methods on simulated data and real EEG signal. We want to prove with the help of an expert the possibility to use these functions on real EEG signal. The results show that the silhouette method applied on EEG recordings is more time-consuming than the elbow method. Neither of the methods is able to correctly recognize the number of clusters in the EEG record by expert evaluation and therefore it is not applicable to the automatic classification of EEG based on k-means algorithm.
Keywords:
silhouette, elbow method, EEG, k-means, automatic determination of number of clusters
Autoři:
Jan Štrobl 1,2; Marek Piorecký 1,2; Vladimír Krajča 1
Působiště autorů:
Faculty of Biomedical Engineering, Czech Technical University in Prague
Kladno, Czech Republic
1; National Institute of Mental Health, Klecany, Czech Republic
2
Vyšlo v časopise:
Lékař a technika - Clinician and Technology No. 3, 2017, 47, 81-87
Kategorie:
Původní práce
Souhrn
Lots of brain diseases are recognized by EEG recording. EEG signal has a stochastic character, this stochastic nature makes the evaluation of EEG recording complicated. Therefore we use automatic classification methods for EEG processing. These methods help the expert to find significant or physiologically important segments in the EEG recording. The k-means algorithm is a frequently used method in practice for automatic classification. The main disadvantage of the k-means algorithm is the necessary determination of the number of clusters. So far there are many methods which try to determine optimal number of clusters for k-means algorithm. The aim of this study is to test functionality of the two most frequently used methods on EEG signals, concretely the elbow and the silhouette method. In this feasibility study we compared the results of both methods on simulated data and real EEG signal. We want to prove with the help of an expert the possibility to use these functions on real EEG signal. The results show that the silhouette method applied on EEG recordings is more time-consuming than the elbow method. Neither of the methods is able to correctly recognize the number of clusters in the EEG record by expert evaluation and therefore it is not applicable to the automatic classification of EEG based on k-means algorithm.
Keywords:
silhouette, elbow method, EEG, k-means, automatic determination of number of clusters
Zdroje
[1] Krajča, V., Mohylová, J.: Číslicové zpracování neurofyzio-logických signálů. V Praze: České vysoké učení technické, 2011, ISBN 9788001047217.
[2] Faber, J.: Elektroencefalografie a psychofyziologie. Praha: ISV, 2001, Lékařství. ISBN 8085866749.
[3] Bizopoulos, P. A., Tsalikakis, D. G., Tzallas, A. T., Koutsouris, D. D., Fotiadis, D. I.: EEG epileptic seizure detection using k-means clustering and marginal spectrum based on ensemble empirical mode decomposition. In: 13th IEEE International Conference on BioInformatics and BioEngineering. Chania: IEEE, 2013, pp. 1–4. DOI: 10.1109/BIBE.2013.6701528.
[4] Krajča, V., Petránek, S.: "Wave-Finder": a new system for an automatic processing of long-term EEG recordings. Quantitative EEG Analysis - Clinical Utility and New Methods. 1993, pp. 103–106.
[5] Värri, A.: Algorithms and systems for the analysis of long-term physiological signals. Tampereen teknillinen korkeakoulu. Julkaisuja. Tampere University of Technology, 1992.
[6] Piorecký, M.: Automatic classification of EEG segments using DBSCAN algorithm. Master thesis. Faculty of Biomedical Engineering, CTU, 2016.
[7] Pollard, K. S., Van der Laan, M. J.: A method to identify significant clusters in gene expression data. Proceedings, SCI World Multi-conference on Systemics, Cybernetics and Informatics, 2002, pp. 318–325.
[8] Frahling, G., Sohler, C.: A fast k-means implementation using coresets. International Journal of Computational Geometry & Applications. 2008, pp. 605–625.
[9] Chiang, M. M.-T., Mirkin, B.: Intelligent Choice of the Number of Clusters in K-Means Clustering: An Experimental Study with Different Cluster Spreads. Journal of Classification. 2010, pp. 3–40. DOI: 10.1007/s00357-010-9049-5.
[10] Sayli, A., Alkan, A. D., Aydin, M.: Determination of relational classification among hull form parameters and ship motions perfor-mance for a set of small. 2016, pp. 1–15. DOI: 10.21278/brod67401.
[11] Azar, A. T., El-Said, S. A., Hassanien, A. E.: Fuzzy and hard clustering analysis for thyroid disease. Computer Me-thods and Programs in Biomedicine. 2013, pp. 1–16. DOI: 10.1016/j.cmpb.2013.01.002.
[12] Ghayekhloo, M., Ghofrani, M., Menhaj, M. B., Azimi, R.: A novel clustering approach for short-term solar radiation forecasting. Solar Energy. 2015, vol. 122, pp. 1371–1383 DOI:10.1016/j.solener.2015.
10.053.
Štítky
BiomedicínaČlánok vyšiel v časopise
Lékař a technika
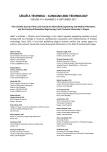
2017 Číslo 3
Najčítanejšie v tomto čísle
- DEVELOPMENT OF NEW TECHNIQUE FOR ACCURATE WEAR ANALYSIS OF EXPLANTED TOTAL HIP REPLACEMENTS
- DESIGN OF AN EXPERIMENTAL LASER SPECKLE CONTRAST IMAGING SYSTEM AND IMAGE
- RELIABILITY AND SOURCE OF ERRORS IN END-TIDAL GAS CONCENTRATION EVALUATION ALGORITHMS DURING AVALANCHE SNOW AND REBREATHING EXPERIMENTS
- DETERMINATION OF THE GEOMETRICAL AND VISCOELASTIC PROPERTIES OF SCAFFOLDS MADE BY ADdITIVE MANUFACTURING USING BIOPLOTTER